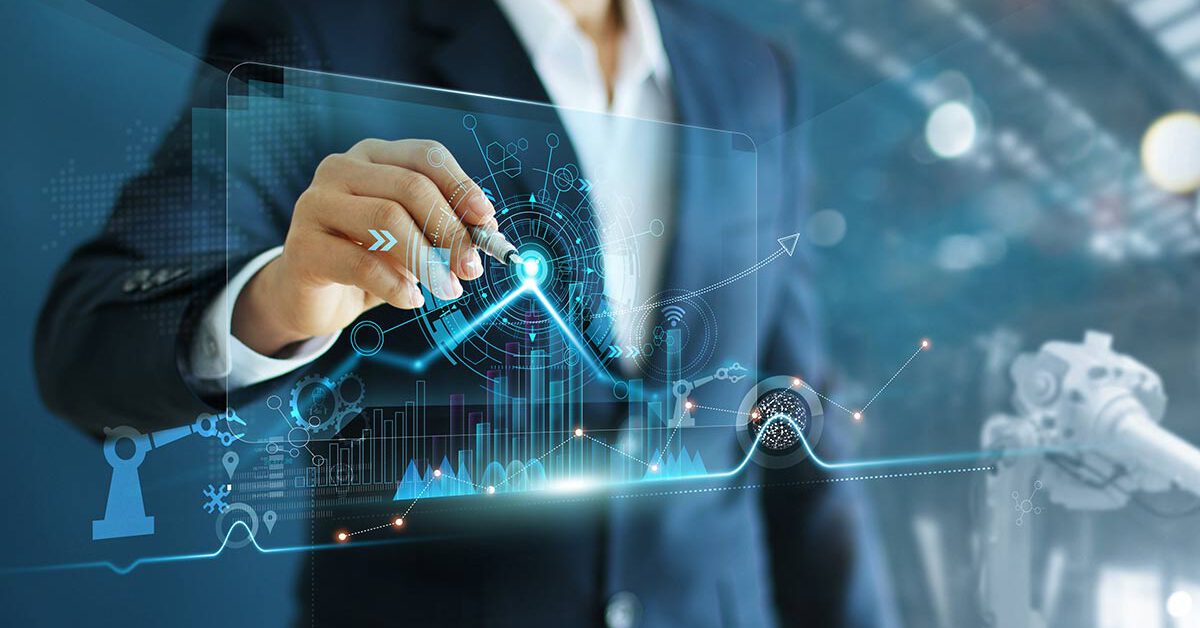
We recently talked about various approaches to asset maintenance and how condition-based methods can help manufacturers minimize unplanned downtime, extending the life of assets while also controlling costs. Now, let’s dive back in and talk about practical steps to implementing a condition-based maintenance program to maximize product uptime.
Common barriers to success
Condition monitoring is great starting point, especially for companies that are still using preventive or scheduled maintenance programs. Rules-based approaches, work well for simple failures—things like predicting when a filter may need to be replaced. But for more complex systems, such as the internal components of an engine, we’ve found that OEMs face two main challenges.
For starters, designing these rules requires a really deep level of domain knowledge surrounding both the physics of the failure mechanism and the design specifications of the equipment. This can be a significant barrier, especially for systems or components that come from a supplier—you simply won’t have access to that proprietary information. The second challenge is that this approach can be difficult to scale. In practice, it’s very rare that equipment is operated within what we might call the “ideal” design specs—although design and engineering teams often set these specs knowing that equipment will be operated in a wide variety of environmental and operating conditions.
Differences in manufacturing and maintenance processes as well as the quality of various components coming from different suppliers can all impact performance. With each machine’s normal baseline performance varying, how do we set accurate thresholds? If you set the threshold close to what the design limits are, you’ll probably get the desired early warning for machines operating close to those limits. But for assets operating farther from that ideal level, you could end up with an unacceptable level of false alarms. On the other hand, if you set the thresholds wider to account for the low performing machines, by the time your best performing machines hit them, it might be too late to prevent a failure.
The alternative is to come up with a unique threshold for each machine. But when you’ve got tens or even hundreds of thousands of machines all running in different conditions, where you simply have too many different permutations and combinations to manage, especially when you also add differences in geography, seasons or climates, age, usage and maintenance practices. For example, think about the difference between a truck operating in the Arizona desert, predominantly delivering goods in the stop and go traffic of the urban areas of Phoenix, tothe one that delivers materials to the Alaskan oil fields during the winter months, running on the frozen Alaskan highway at a steady speed carrying very heavy loads. If you apply the desert truck’s coolant temperature thresholds to the one in Alaska, it would be too late to prevent a failure by the time that alert goes out. That’s why a multi-variate model-based approach – for e.g. anomaly detection, is a more effective approach that’s also easier to scale.
Putting proactive maintenance into practice
Let’s start with the obvious implementation requirements, which are table stakes. You need to be connected to your products in the field via an IoT platform or telematic service provider, with sensors delivering a good quantity (and quality) of sensor data. Sensor data needs to be streaming continuously and in near real time, taking a snapshot every few minutes at least. You need a place to store all that data, such as a data lake. And historic data is a prerequisite for training the machine learning models—six months is good, 12 is better.
Here’s where many organizations run into trouble. You have the sensors, and the data, and maybe you’ve even hired data scientists to analyze it and build, train, and test machine learning models. But how do you move from the lab environment into the real world? In other words, how do you operationalize your program and turn it into a business service offering for your customers in a way that’s sustainable and delivers ROI? Do you have the right organizational structure in place to monitor all of your connected assets in the field? What types of subject matter experts do you need? Once you find a potential issue with one of your assets, what’s the workflow to resolve it?
While analytics and data science are key, what companies often don’t realize is that domain knowledge is even more important. The machines we’re dealing with are designed based on physics and engineering principles. Understanding what’s important to model, what is and isn’t feasible, where to focus—all this information comes from the business and domain side, not from data science. That’s why taking a siloed approach won’t work. You need to bring together the technical people who know how to analyze and model the data and the domain experts who understand what that data means in the real world, out in the field. Even if you manage to operationalize the analytics to automatically process the near real-time data, you need to train the analysts and technicians – workforce typically trained to act on a customer reported issue – to interpret the anomaly and failure notifcations, proactively notify and convince the customer to schedule maintenance at the appropriate time. Working out these business processes and organizational changes is a crucial piece of the puzzle that most organizations fail to pay enough attention to.
IT considerations
It’s important to choose a technology stack and build a UI that ensures you can continually enhance and maintain the predictive maintenance system over time as your business evolves and your needs change. Factoring in the time and cost involved with building this kind of solution from the ground up, an off-the-shelf solution is likely to be a better investment. You can still build your value-added services on top—whether simple monitoring and alerts, or more sophisticated anomaly detection and failure prediction. Depending on your service offering and monetization strategy, you may need to add additional functionality to support your services, such as case management to capture the expert knowledge required to translate the failure symptoms to a root cause and corrective actions and ultimately turn this into a curated knowledge base for the time after your experienced baby boomer generation expert has retired, etc.
You’ll also need a solution that integrates easily with all your existing data sources and systems—everything from sensor or telematics data to service history and maintenance planning systems. Bridging the gap between data science and domain expertise ensures that the people who can understand the root cause of an issue and know how to fix it will be able to visualize and analyze any model-based alerts or notifications without the need for coding or software skills, and in the process allow for seamless communication between your data scientists, business analysts, customer support teams, dealer technicians and the customerIf the model isn’t clear, you may need to schedule a manual inspection to verify the problem before you decide what steps to take, especially if the component that may need replacing is costly. This is a holistic business process involving multiple organizations and systems, and requires the breaking down of silos with a system that’s accessible by more than a small group of people with a specific skillset.
Whereas software developers are used to pushing out new products or updates every quarter if not faster, in manufacturing we measure new product introduction (NPI) in years. This makes recruiting a software team to work in your manufacturing company a real challenge. Not only are the skills different, the culture and mindset are different, too. Many OEMs find more success by building an ecosystem of partners that bring the right skills to the table, rather than trying to build a solution from the ground up. This even applies to manufacturing giants like GE, which spent years trying to build its own APM platform without much success.
For OEMs, the best path forward is to focus on your core strength which is a deep understanding of your business, your machines, and your customers. Partnering with the right predictive maintenance solution provider and bringing your own knowledge to their technology will allow you to accelerate time to value and time to journey. That’s the way to succeed.
If you’re ready to put your sensor data to work and power more product uptime, Syncron can help. Head to our Syncron Uptime™ product page to get started.